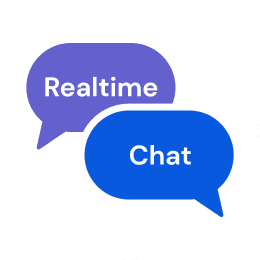
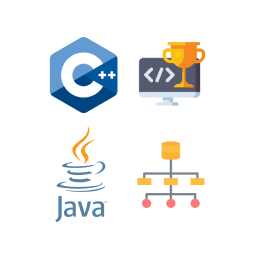
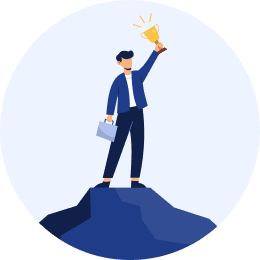
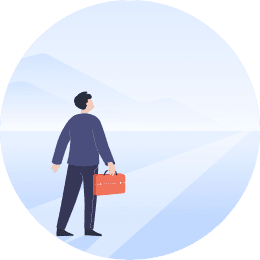
Share it on social media and in your performance review
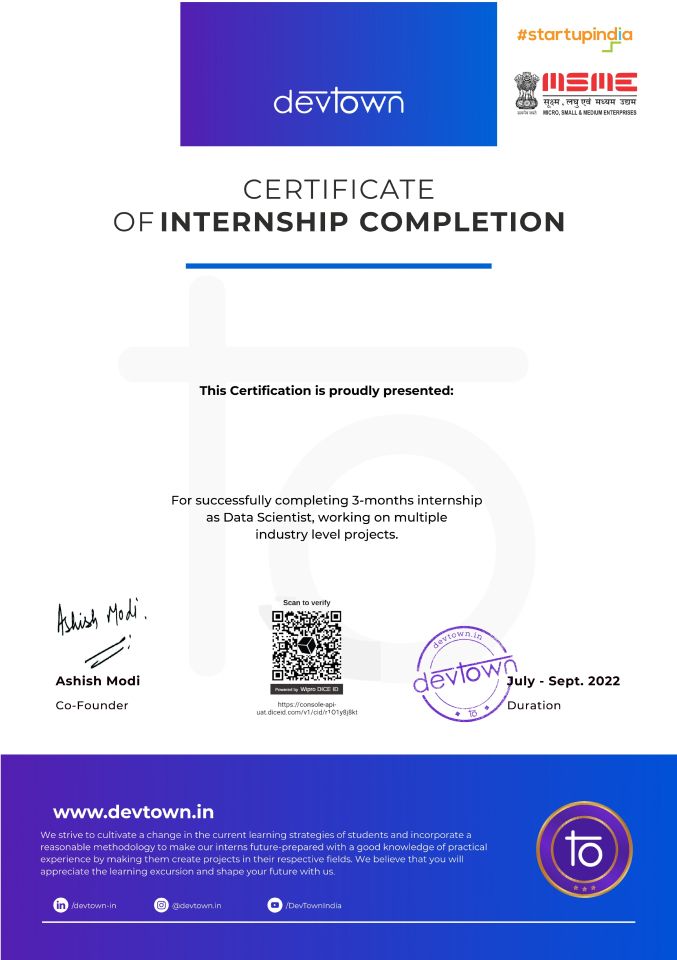
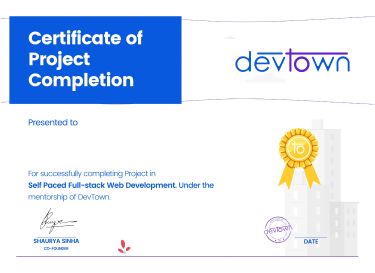
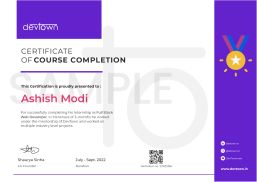
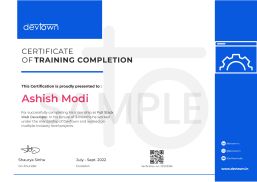

















Introduction to PythonModule 120 Hours3 Projects
- Data Types and Operators: Understand Python's fundamental data types and operators.
- Control Flow: Learn about conditional statements and loops.
- Scripting: Develop scripts for automating tasks using Python.
- Object-Oriented Programming (OOP) in Python: Master OOP concepts such as classes and inheritance.
Introduction to Data AnalysisModule 220 Hours1 Projects
- Data Analysis Process: Learn the step-by-step process of data analysis.
- Python Tools for Data Analysis: Dive into data analysis libraries like NumPy and Pandas.
- Data Plotting: Use Matplotlib and Seaborn for data visualization.
Statistics for Data AnalysisModule 330 Hours2 Projects
- Constructs: Understand basic statistical constructs.
- Population vs Sample: Differentiate between population and sample statistics.
- Correlation vs Causation: Learn about the difference between correlation and causation.
- Hypotheses: Formulate hypotheses for statistical testing.
- Experimentation: Understand experimental design and hypothesis testing.
- Visualizing Data: Use Python libraries like Matplotlib and Seaborn for data visualization.
- Central Tendency and Variability: Explore measures of central tendency and variability.
- Standardizing and Sampling Distribution: Learn about standardization and sampling distributions.
- Estimating and Hypothesis Testing: Perform hypothesis testing and parameter estimation.
- T-Tests: Conduct T-tests for comparing means.
SQL for Data AnalysisModule 440 Hours2 Projects
- Basic SQL: Learn SQL syntax and commands.
- SQL Join: Understand different types of SQL joins for combining data.
- Aggregations: Perform aggregations using SQL functions.
- Subqueries & Temporary Tables: Use subqueries and temporary tables for complex queries.
- SQL Data Cleaning: Clean and preprocess data using SQL.
- Window Functions: Utilize window functions for advanced data analysis.
- SQL Advanced JOINs: Explore advanced JOIN operations.
- Performance Tuning: Optimize SQL queries for better performance.
- Accessing Database using Python: Connect Python applications to databases using SQL.
Introduction to Machine LearningModule 510 Hours2 Projects
- Linear Regression: Understand simple, multiple, and polynomial linear regression.
- Classification Algorithms: Explore logistic regression, decision trees, and K-nearest neighbors.
- Clustering Algorithms: Learn about K-means and hierarchical clustering.
- Association Rule Learning: Study Apriori and Eclat algorithms for market basket analysis.
- Natural Language Processing (NLP): Dive into NLP techniques using Python libraries.
Introduction to Deep LearningModule 610 Hours1 Projects
- Introduction to Deep Learning: Learn the basics of deep neural networks using Keras, TensorFlow, and PyTorch.
- Artificial Neural Networks (ANN): Understand the architecture and training of ANNs.
- Convolutional Neural Networks (CNN): Learn CNNs for image recognition tasks.
- Recurrent Neural Networks (RNN): Explore RNNs for sequential data analysis.
- Autoencoders and GANs: Study autoencoders and generative adversarial networks.
- Natural Language Processing (NLP): Apply deep learning techniques to NLP tasks.
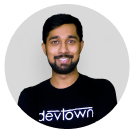
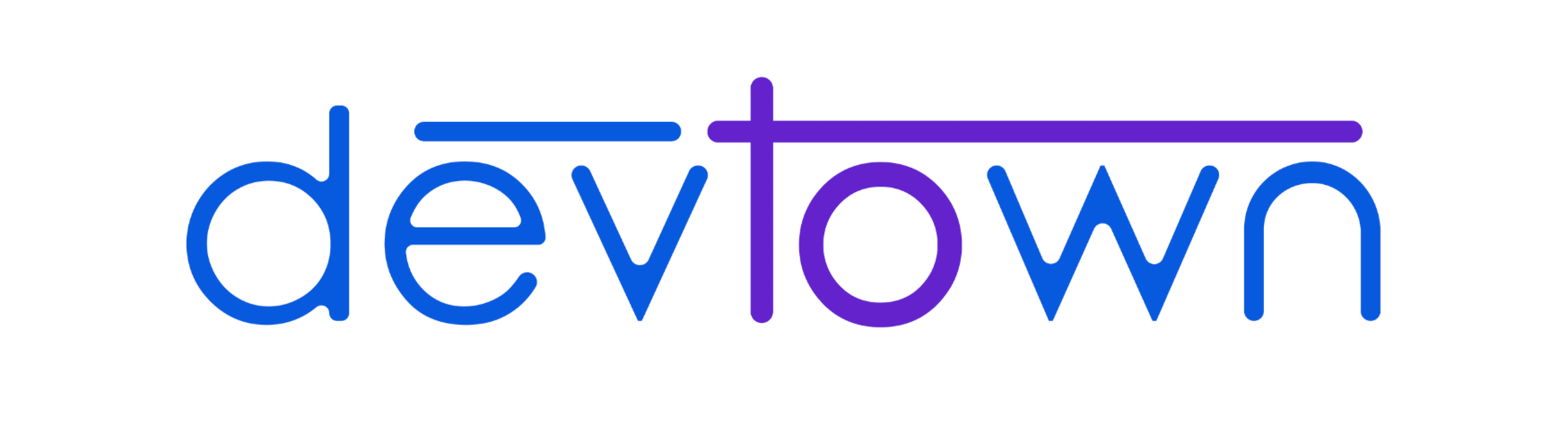
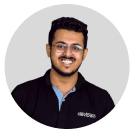
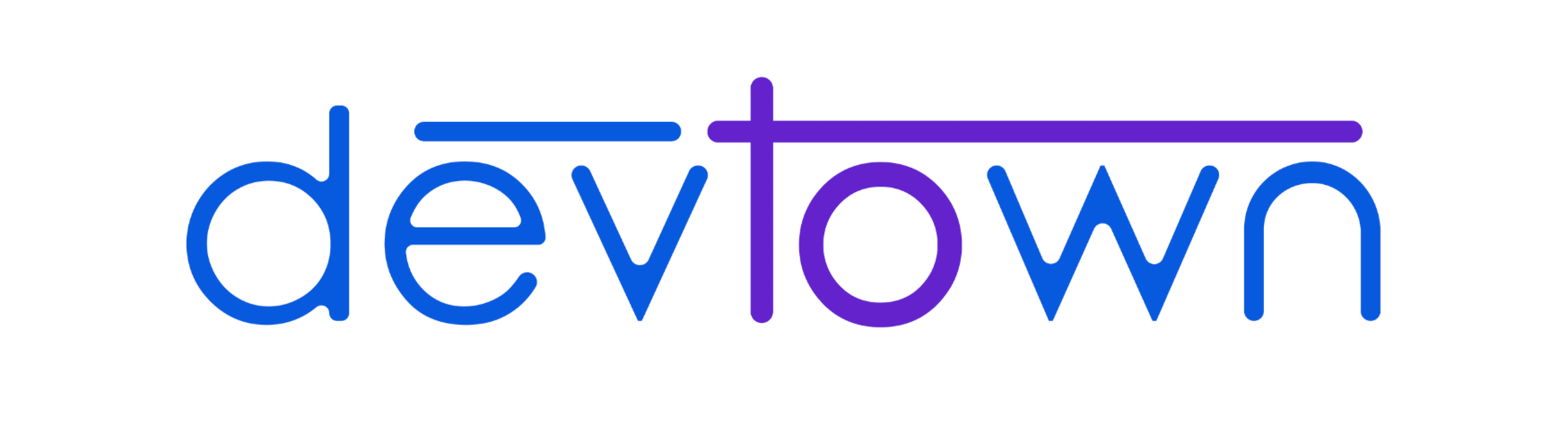
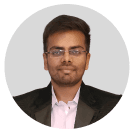
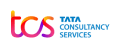
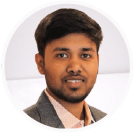
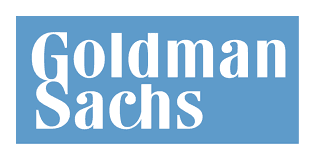
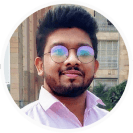
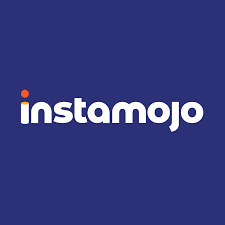
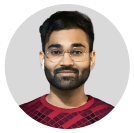
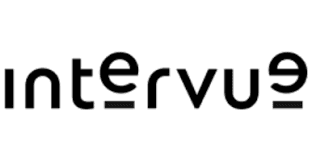
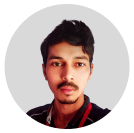
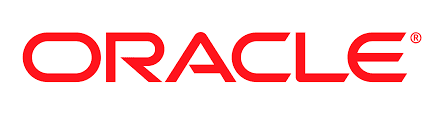
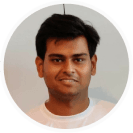
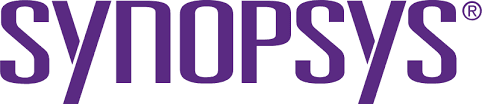
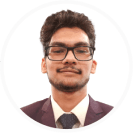
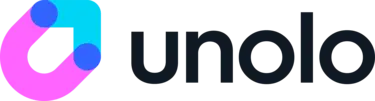
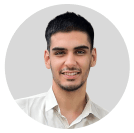
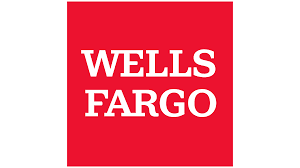
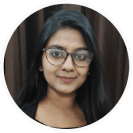
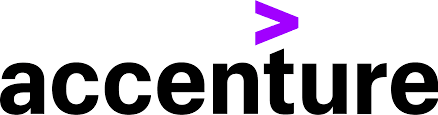
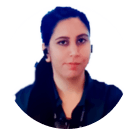
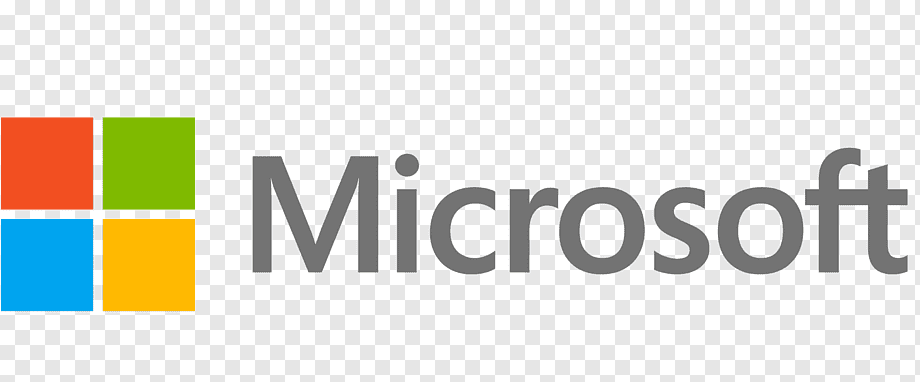
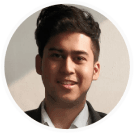
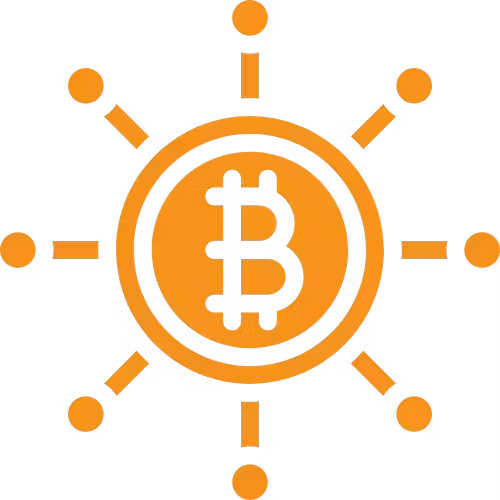
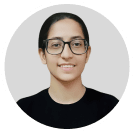
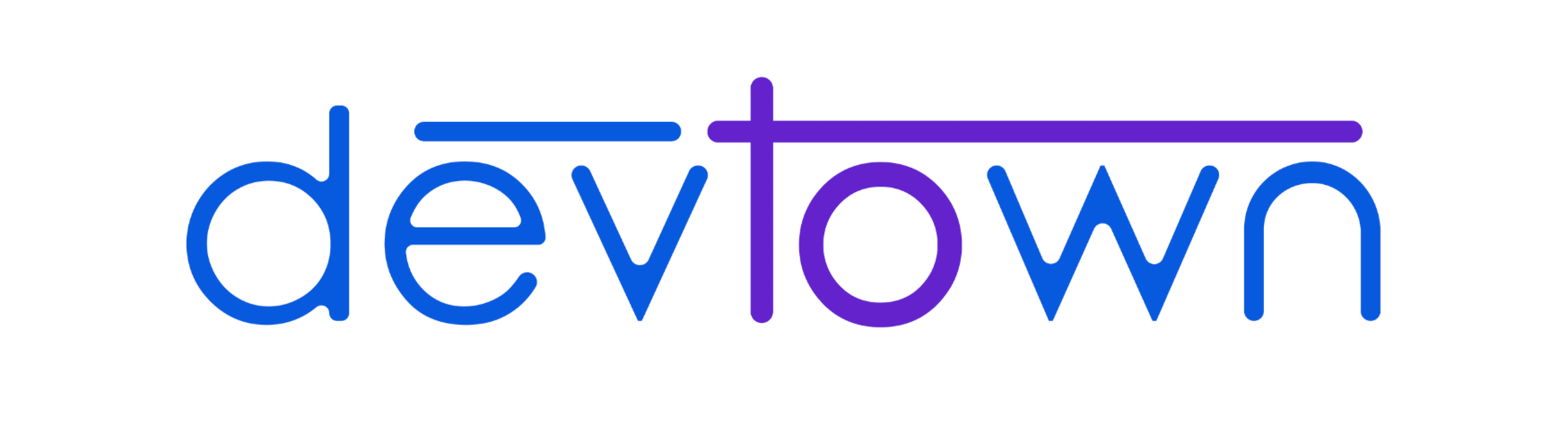
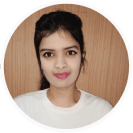
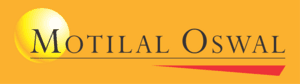
What is the hype around free complimentary courses?
In addition to your main domain course, you'll receive access to four complimentary courses at no extra cost. These include courses on DSA, CP, C++ JAVA, which hold significant value in enhancing your problem-solving abilities and preparing you for technical interviews. We provide these courses for free with lifetime access, ensuring that you have ample resources to enhance your skills and succeed in your learning journey.
Are there any prerequisites for enrolling in this course?
No, there aren't any prerequisites. Our courses are open to individuals from various academic backgrounds, including Mathematics, Statistics, Computer Science, Engineering, Business, and more. Many successful learners have transitioned into rewarding data science careers without any specific prior requirements.
What if I find it challenging to keep up with the pace of the course?
We understand that everyone learns at their own speed. With our self-paced course, you have the flexibility to progress through the material at your own pace.
Will I have access to the course material after completing it?
Yes, you will have lifetime access to the course material, allowing you to revisit it whenever needed to reinforce your learning. Additionally, along with the main course, you will also have access to complimentary courses for lifetime, further enriching your learning experience.
What about the projects I'll be working on?
You'll have the opportunity to work on 10+ live projects covering various aspects of data science, including predictive modeling, data visualization, and machine learning. Projects may involve analyzing real-world datasets, building predictive models, and deriving actionable insights. Depending on your batch, you'll be assigned to work on any two projects during your journey. Towards the end of the program, you'll undertake a capstone project where you'll apply your skills to solve a real-world data science problem, showcasing your proficiency to potential employers.
Is it like any other course, or do I really get to learn everything from scratch?
Trust us, this program covers everything you need to know about data science. You'll embark on a journey from the basics to advanced concepts, gaining comprehensive knowledge along the way. However, it's important to recognize that learning is a lifelong pursuit. While this program will equip you with the skills and projects needed to enter the data science field confidently, continuous self-improvement is key. The projects you complete and the knowledge you gain during this program will undoubtedly prepare you for the data science industry, but remember, learning is an ongoing process that extends beyond the confines of any course.